Statistics Crash Course: 5 Basic Tests and How to Use Them
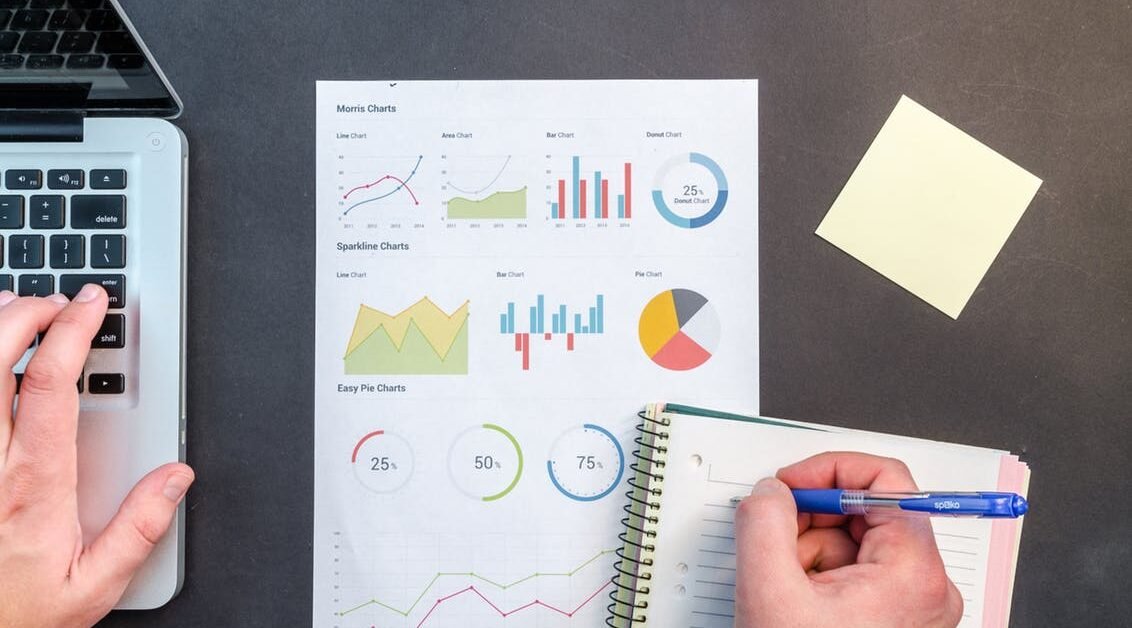
Statistics are a critical cog in the research machine. Whether you’re trying to find actionable results in your dataset or trying to figure out the details of a report, knowing about the most common statistical methods and how they are applied is the gateway to understanding.
Here are five of the most common statistical analyses used in modern research, what they are, and when they are used:
- Descriptives
- What they are: Numbers that describe a dataset.
- When to use them: When you want to get a general sense of your findings, and how much agreement there was across participants.
- The details: Descriptives include measures of central tendency such as the mean (the average), median (middle-point), and mode (the most common point), as well as measures of variability (how spread out your data is) such as range and standard deviation. While the math behind these is much simpler than the other statistical tests on this list, it is likely the most prevalent type of analysis in all of statistics. There is a rather simple reason for that, too. Descriptive analyses tell you what your data look like, and as such, it is frequently a first step (or a simultaneous step if you are using programs like SPSS) when doing data analysis. Gaining a general sense of how your participants responded as a whole can help you understand the results of some of the other tests you run. It can also help you understand how much (or how little) your participants agreed with each other.
- T-test
- What it is: A test for comparing the averages of two groups.
- When to use it: When you want to see if two groups are actually distinct from each other, or if the same group differs at two separate points in their experience or with two different devices.
- The details: The T-test is the most basic way to see if two groups are “significantly” different from one another. It is used in null hypothesis testing, wherein the goal is essentially to determine if your hypothesis (what you think is happening) exists or not. Basically, you take a measurement of two groups (e.g., one group using one device and another group using another device) under the assumption that there are no differences between them. The T-test will tell you if there is a large enough difference between the two to doubt that assumption, and if that is the case, you can reject the assumption (or null hypothesis).
- ANOVA
- What it is: A test that reveals the variance between multiple variables.
- When to use it: When you have a complex study that involves multiple groups, multiple measures over time, or both, and you want to know if there are differences between those groups or over time.
- The details: The ANOVA, or ANalysis Of VAriance, is one of the more common statistical tests used in research. As the name implies, the ANOVA is a comparison of different means (typically 3+) to determine whether or not they are different. ANOVA is comparable to the t-test, which compares two variables. In fact, assuming your groups are equal, you may even be able to substitute a bunch of t-tests for an ANOVA. The complication may leave you with error, however, so it’s probably best to stick with the ANOVA. There is a wide variety of ways to run an ANOVA, so it is important to know what your study entails before running this test. Knowing the number of independent variables (e.g., the number of devices being tested) and whether you are running a within-subjects (e.g., every participant uses every device) or between-subjects (e.g., half of the participants use one device and half use another) study is particularly important. If used properly, the ANOVA can be a powerful analytical tool.
- Repeated measures
- What it is: A test to analyze measurements of the same variable at multiple points in time.
- When to use it: When you want to examine changes in a group over time (e.g., a longitudinal diary study), particularly if you are intervening (e.g., with a device, a service, training, etc.) and want to see if that intervention has an actual influence on the group.
- The details: The statistical test for repeated measures is a specific subset of ANOVA, often called rANOVA (think ‘r’ for ‘repeated’). It employs a mixture of within-subjects and between-subjects designs in order to understand how interventions or other variables can influence groups over time. For example, a simple repeated measures study may have a control group and a test group. Both groups are measured at the start, then the test group alone is given some sort of intervention (they are experience your product or undergo some treatment), then both groups are again measured. The rANOVA looks at the differences between the measurements taken to determine if the intervention had any true effects. This test has a broad range of applicability, and as such is one of the most commonly used tests.
- Regression
- What it is: A test for estimating how variables relate to each other and to potential outcomes.
- When to use it: When you want to predict the future.
- The Details: In essence, regression is simply a technique used to estimate how variables relate to one another and how they each influence the outcome. Like the ANOVA, regression can come in many forms, so before you attempt to use it, it is important to understand your research goals. Regression can be linear or nonlinear, and can have just one input or many. Perhaps the most common use of regression is in linear regression, which has many practical uses including being able to create predictive models. Let’s look at weather as an example. If you wanted to determine whether it is going to rain or not tomorrow, you might look at temperature, pressure, humidity, cloud cover, and historical weather pattern information. Each of those variables will have a different influence in ultimate outcome. For instance, cloud cover may influence tomorrow’s rain potential more than historical data or temperature. Using regression, we can figure out how strongly each variable relates to the ultimate outcome. Coupling that with current data we have an estimate of the likelihood of rain tomorrow. The same methods can be used to predict stock prices, election outcomes, or just about anything else. Very useful stuff when used correctly.
There are many other statistical tests and methods out there, but knowing at least what these five tests are and when they are used is a great starting point for understanding reports and data. This can also be a starting point for decision making when it comes to your own research. Fitting the proper statistical tests to your project early in its development will help guide the research design and set you up for success. However you apply this knowledge, these are five statistical tests you’ll be glad you know.
At Human Interfaces, an expert team of UX professionals work with clients from a variety of industries to develop custom research solutions for any UX challenge. If you need help from a full-service UX research consultancy for a study, recruitment, or facility rental, visit our website, send us an email, or connect with us through LinkedIn.